Screening challenges | Part 2 - Applying data management concepts to watchlist data
Screening challenges | Part 2 - Applying data management concepts to watchlist data


Deep Pai
11 Mar 2024
Sanctions Screening
Sanctions Screening
Sanctions Screening
Sanctions Screening
Sanctions Screening
In the first part of the series “Continuous screening challenges”, we looked at automating watchlist data operations using a good watchlist management solution to increase the speed of compliance. In this next blog, we focus on principles of data quality management to improve screening outcomes.
Compliance teams must monitor and sift through multiple global and local sanctions watchlists to ensure timely regulatory compliance. Unfortunately, they find themselves in the position where their screening software is simply generating too many false positives due to poor quality of watchlist data. This in turn results in a lack of trust and a poor compliance culture, where alerts are not assessed correctly, eventually leading to the possibility of criminal activity being overlooked. The outcome of this can be significant compliance and risk exposure – including regulatory reprimands, financial penalties, and adverse media headlines.
The importance of data quality management
The Data Management Association (DAMA) defines data quality management as the “Planning, implementation and control of activities, to assure that data assets are fit for consumption and meet the needs of data consumers.” Having the right data quality management tools and processes is of vital importance for managing watchlists successfully. For regulated entities and businesses, these tools and processes can make the difference between meeting screening compliance obligations and failing to do so.
Data quality management is composed of many elements, such as:
Accuracy – The correctness of the data.
For watchlist data, this can be how well the data reflects the entities and individuals meant to be monitored by the list, as well as how accurate the data is compared with the information originally provided by the data sources.
Owner – Who owns the watchlist data.
Watchlist data can have an external owner – for example, a government that publishes a sanctions list. It also should have an internal owner – the individual responsible for the use of the data provided by the organisation.
Lineage – This is the process of tracking the flow of data over time.
Lineage should provide information about where the data originated, how it has changed, and how it is being used. Understanding lineage is of growing importance for watchlist management as regulators want to understand how data is used in decision-making.
Timeliness – How fast the data is updated.
Today regulators want to see watchlist data updated in minutes, not days. It is important that watchlist data is delivered to your screening engines as soon as they are updated.
Consistency – Uniform file formats.
Watchlist data needs to be in correct data formats and structures to ensure that it is processed by screening software correctly across the organisation. Watchlist data can come from many sources and can be used in multiple systems. So, it is essential that the data from multiple sources is available in necessary formats for consumption.
Completeness – Ensuring that all mandatory data is present in the watchlist.
Sometimes, fields such as birthdays or places of birth may be incomplete or missing. However, having data as complete as possible reduces false positives in screening.
These various elements of data quality should be tracked within a watchlist management solution using metadata.
Understanding metadata
According to Gartner, metadata is information that describes various elements of an information asset to improve its usability throughout its life cycle. It unlocks the value of data by helping to answer “what, where, when, how, and who” of the data. Sometimes, it is also described as “data about data”. Metadata comes in two forms:
Technical metadata provides information such as data storage and structure and other elements such as data attributes, data lineage and access credentials.
Business metadata delivers business context. It defines everyday business terms and rules, as well as information about data ownership, classifications, and relationships.
A watchlist data management solution should actively manage and use both technical and business metadata to provide users with the information about the quality of the data being used in screening processes.
Delivering explainability
Data quality and metadata are best managed within a data governance framework. To begin with, this means having a single watchlist. Many financial firms today will have multiple watchlists. By using multiple lists, firms often hope to ensure compliance, with the strength of one list making up for the deficit of another list. However, usually the data is not properly managed creating errors. Screening against multiple lists also takes more time and increases the number of false positives. Updating these lists can sometimes take up to 12 to 24 hours, resulting in potential sanctions breaches. Also, using multiple lists often means firms cannot explain how they have arrived at their decisions.
Instead, firms should have a single watchlist managed by a watchlist data management solution that has a built-in data governance framework. This framework should be able to use both business metadata and technical metadata to deliver explainability, i.e. how the watchlist data has been used by the screening system to make decisions. The watchlist data management solution should deliver this information through clear and concise reporting, facilitating comprehension for compliance teams and seamless provision to regulators upon request. Having robust data governance also enables compliance teams to proactively manage data quality, reducing false positives, operational risks and compliance risks within screening.
Conclusion
Effective management of watchlists requires proper data quality tools and processes. A watchlist data management solution should provide automation and support screening to compliance teams and proactively manage data quality with a single watchlist. This watchlist must be managed within a data governance framework. Only robust data management can support high data quality and help reduce compliance exposure and risk.
Stay tuned for our next blog where we will cover Master Data Management - the third challenge of a screening solution.
To find out how FacctList can revolutionise your watchlist management, write to us at sales@facctum.com
In the first part of the series “Continuous screening challenges”, we looked at automating watchlist data operations using a good watchlist management solution to increase the speed of compliance. In this next blog, we focus on principles of data quality management to improve screening outcomes.
Compliance teams must monitor and sift through multiple global and local sanctions watchlists to ensure timely regulatory compliance. Unfortunately, they find themselves in the position where their screening software is simply generating too many false positives due to poor quality of watchlist data. This in turn results in a lack of trust and a poor compliance culture, where alerts are not assessed correctly, eventually leading to the possibility of criminal activity being overlooked. The outcome of this can be significant compliance and risk exposure – including regulatory reprimands, financial penalties, and adverse media headlines.
The importance of data quality management
The Data Management Association (DAMA) defines data quality management as the “Planning, implementation and control of activities, to assure that data assets are fit for consumption and meet the needs of data consumers.” Having the right data quality management tools and processes is of vital importance for managing watchlists successfully. For regulated entities and businesses, these tools and processes can make the difference between meeting screening compliance obligations and failing to do so.
Data quality management is composed of many elements, such as:
Accuracy – The correctness of the data.
For watchlist data, this can be how well the data reflects the entities and individuals meant to be monitored by the list, as well as how accurate the data is compared with the information originally provided by the data sources.
Owner – Who owns the watchlist data.
Watchlist data can have an external owner – for example, a government that publishes a sanctions list. It also should have an internal owner – the individual responsible for the use of the data provided by the organisation.
Lineage – This is the process of tracking the flow of data over time.
Lineage should provide information about where the data originated, how it has changed, and how it is being used. Understanding lineage is of growing importance for watchlist management as regulators want to understand how data is used in decision-making.
Timeliness – How fast the data is updated.
Today regulators want to see watchlist data updated in minutes, not days. It is important that watchlist data is delivered to your screening engines as soon as they are updated.
Consistency – Uniform file formats.
Watchlist data needs to be in correct data formats and structures to ensure that it is processed by screening software correctly across the organisation. Watchlist data can come from many sources and can be used in multiple systems. So, it is essential that the data from multiple sources is available in necessary formats for consumption.
Completeness – Ensuring that all mandatory data is present in the watchlist.
Sometimes, fields such as birthdays or places of birth may be incomplete or missing. However, having data as complete as possible reduces false positives in screening.
These various elements of data quality should be tracked within a watchlist management solution using metadata.
Understanding metadata
According to Gartner, metadata is information that describes various elements of an information asset to improve its usability throughout its life cycle. It unlocks the value of data by helping to answer “what, where, when, how, and who” of the data. Sometimes, it is also described as “data about data”. Metadata comes in two forms:
Technical metadata provides information such as data storage and structure and other elements such as data attributes, data lineage and access credentials.
Business metadata delivers business context. It defines everyday business terms and rules, as well as information about data ownership, classifications, and relationships.
A watchlist data management solution should actively manage and use both technical and business metadata to provide users with the information about the quality of the data being used in screening processes.
Delivering explainability
Data quality and metadata are best managed within a data governance framework. To begin with, this means having a single watchlist. Many financial firms today will have multiple watchlists. By using multiple lists, firms often hope to ensure compliance, with the strength of one list making up for the deficit of another list. However, usually the data is not properly managed creating errors. Screening against multiple lists also takes more time and increases the number of false positives. Updating these lists can sometimes take up to 12 to 24 hours, resulting in potential sanctions breaches. Also, using multiple lists often means firms cannot explain how they have arrived at their decisions.
Instead, firms should have a single watchlist managed by a watchlist data management solution that has a built-in data governance framework. This framework should be able to use both business metadata and technical metadata to deliver explainability, i.e. how the watchlist data has been used by the screening system to make decisions. The watchlist data management solution should deliver this information through clear and concise reporting, facilitating comprehension for compliance teams and seamless provision to regulators upon request. Having robust data governance also enables compliance teams to proactively manage data quality, reducing false positives, operational risks and compliance risks within screening.
Conclusion
Effective management of watchlists requires proper data quality tools and processes. A watchlist data management solution should provide automation and support screening to compliance teams and proactively manage data quality with a single watchlist. This watchlist must be managed within a data governance framework. Only robust data management can support high data quality and help reduce compliance exposure and risk.
Stay tuned for our next blog where we will cover Master Data Management - the third challenge of a screening solution.
To find out how FacctList can revolutionise your watchlist management, write to us at sales@facctum.com
In the first part of the series “Continuous screening challenges”, we looked at automating watchlist data operations using a good watchlist management solution to increase the speed of compliance. In this next blog, we focus on principles of data quality management to improve screening outcomes.
Compliance teams must monitor and sift through multiple global and local sanctions watchlists to ensure timely regulatory compliance. Unfortunately, they find themselves in the position where their screening software is simply generating too many false positives due to poor quality of watchlist data. This in turn results in a lack of trust and a poor compliance culture, where alerts are not assessed correctly, eventually leading to the possibility of criminal activity being overlooked. The outcome of this can be significant compliance and risk exposure – including regulatory reprimands, financial penalties, and adverse media headlines.
The importance of data quality management
The Data Management Association (DAMA) defines data quality management as the “Planning, implementation and control of activities, to assure that data assets are fit for consumption and meet the needs of data consumers.” Having the right data quality management tools and processes is of vital importance for managing watchlists successfully. For regulated entities and businesses, these tools and processes can make the difference between meeting screening compliance obligations and failing to do so.
Data quality management is composed of many elements, such as:
Accuracy – The correctness of the data.
For watchlist data, this can be how well the data reflects the entities and individuals meant to be monitored by the list, as well as how accurate the data is compared with the information originally provided by the data sources.
Owner – Who owns the watchlist data.
Watchlist data can have an external owner – for example, a government that publishes a sanctions list. It also should have an internal owner – the individual responsible for the use of the data provided by the organisation.
Lineage – This is the process of tracking the flow of data over time.
Lineage should provide information about where the data originated, how it has changed, and how it is being used. Understanding lineage is of growing importance for watchlist management as regulators want to understand how data is used in decision-making.
Timeliness – How fast the data is updated.
Today regulators want to see watchlist data updated in minutes, not days. It is important that watchlist data is delivered to your screening engines as soon as they are updated.
Consistency – Uniform file formats.
Watchlist data needs to be in correct data formats and structures to ensure that it is processed by screening software correctly across the organisation. Watchlist data can come from many sources and can be used in multiple systems. So, it is essential that the data from multiple sources is available in necessary formats for consumption.
Completeness – Ensuring that all mandatory data is present in the watchlist.
Sometimes, fields such as birthdays or places of birth may be incomplete or missing. However, having data as complete as possible reduces false positives in screening.
These various elements of data quality should be tracked within a watchlist management solution using metadata.
Understanding metadata
According to Gartner, metadata is information that describes various elements of an information asset to improve its usability throughout its life cycle. It unlocks the value of data by helping to answer “what, where, when, how, and who” of the data. Sometimes, it is also described as “data about data”. Metadata comes in two forms:
Technical metadata provides information such as data storage and structure and other elements such as data attributes, data lineage and access credentials.
Business metadata delivers business context. It defines everyday business terms and rules, as well as information about data ownership, classifications, and relationships.
A watchlist data management solution should actively manage and use both technical and business metadata to provide users with the information about the quality of the data being used in screening processes.
Delivering explainability
Data quality and metadata are best managed within a data governance framework. To begin with, this means having a single watchlist. Many financial firms today will have multiple watchlists. By using multiple lists, firms often hope to ensure compliance, with the strength of one list making up for the deficit of another list. However, usually the data is not properly managed creating errors. Screening against multiple lists also takes more time and increases the number of false positives. Updating these lists can sometimes take up to 12 to 24 hours, resulting in potential sanctions breaches. Also, using multiple lists often means firms cannot explain how they have arrived at their decisions.
Instead, firms should have a single watchlist managed by a watchlist data management solution that has a built-in data governance framework. This framework should be able to use both business metadata and technical metadata to deliver explainability, i.e. how the watchlist data has been used by the screening system to make decisions. The watchlist data management solution should deliver this information through clear and concise reporting, facilitating comprehension for compliance teams and seamless provision to regulators upon request. Having robust data governance also enables compliance teams to proactively manage data quality, reducing false positives, operational risks and compliance risks within screening.
Conclusion
Effective management of watchlists requires proper data quality tools and processes. A watchlist data management solution should provide automation and support screening to compliance teams and proactively manage data quality with a single watchlist. This watchlist must be managed within a data governance framework. Only robust data management can support high data quality and help reduce compliance exposure and risk.
Stay tuned for our next blog where we will cover Master Data Management - the third challenge of a screening solution.
To find out how FacctList can revolutionise your watchlist management, write to us at sales@facctum.com
Latest blogs
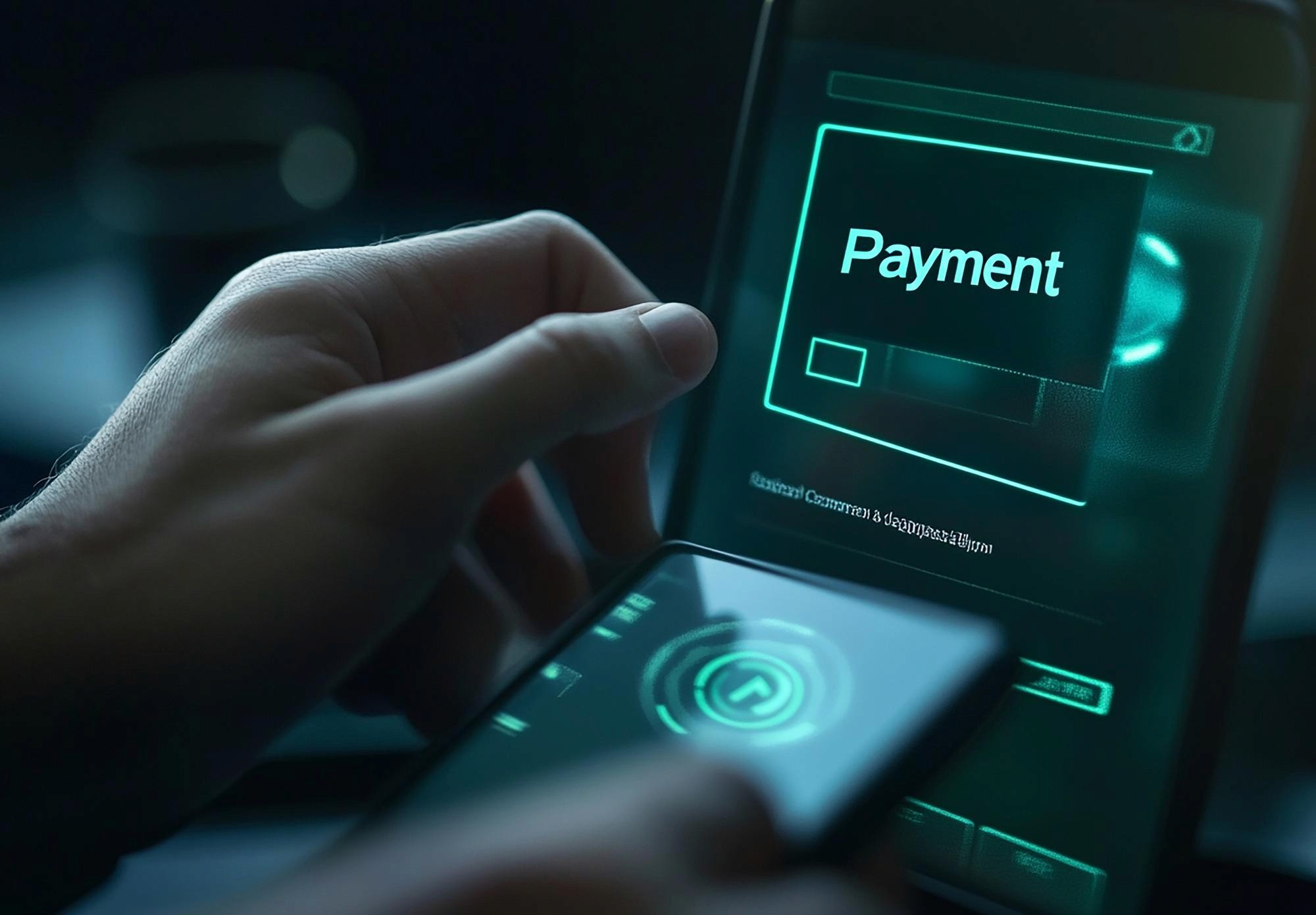
Watchlist Management
SEPA Instant Payments: Transforming Real-Time Transactions
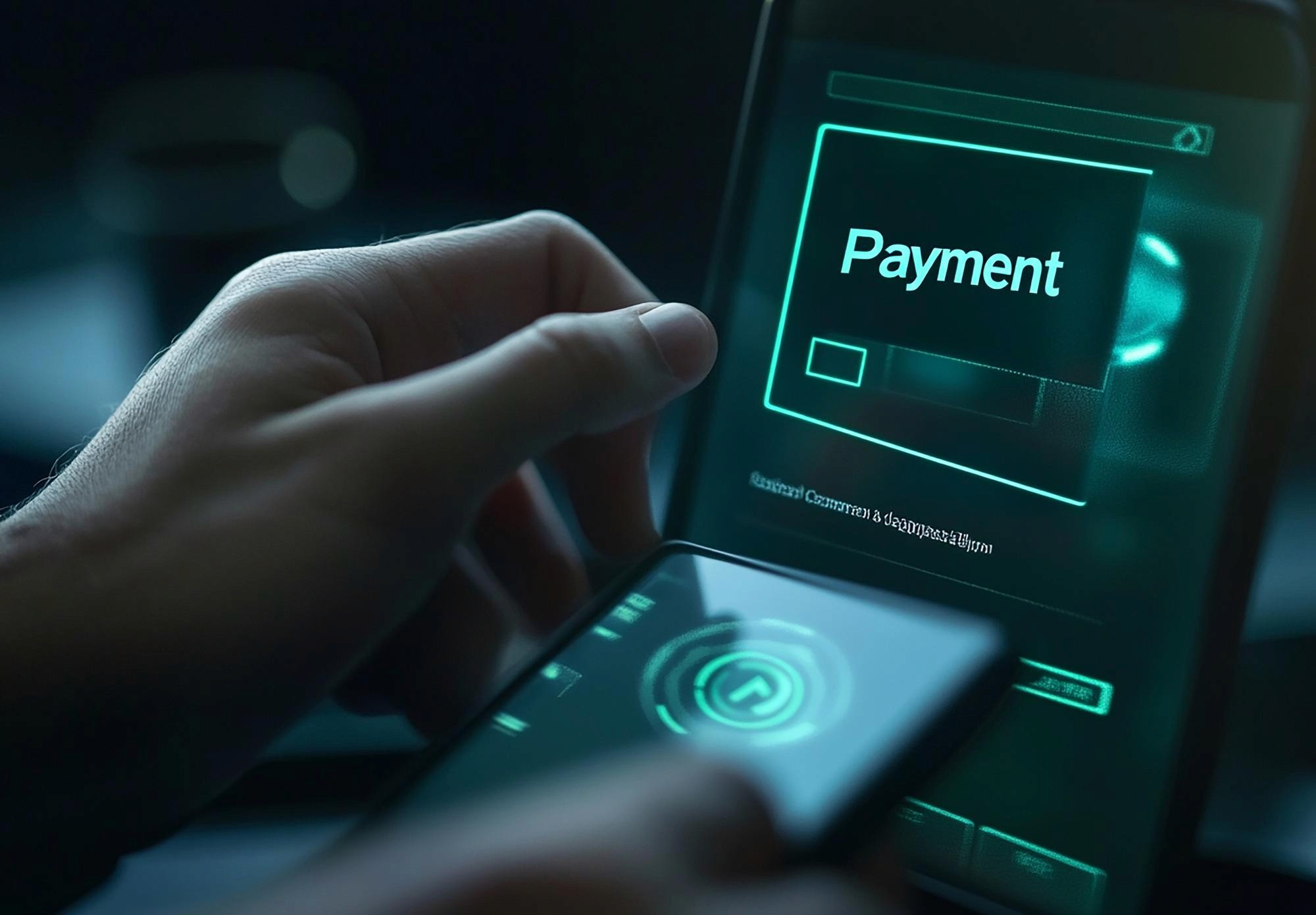
Watchlist Management
SEPA Instant Payments: Transforming Real-Time Transactions
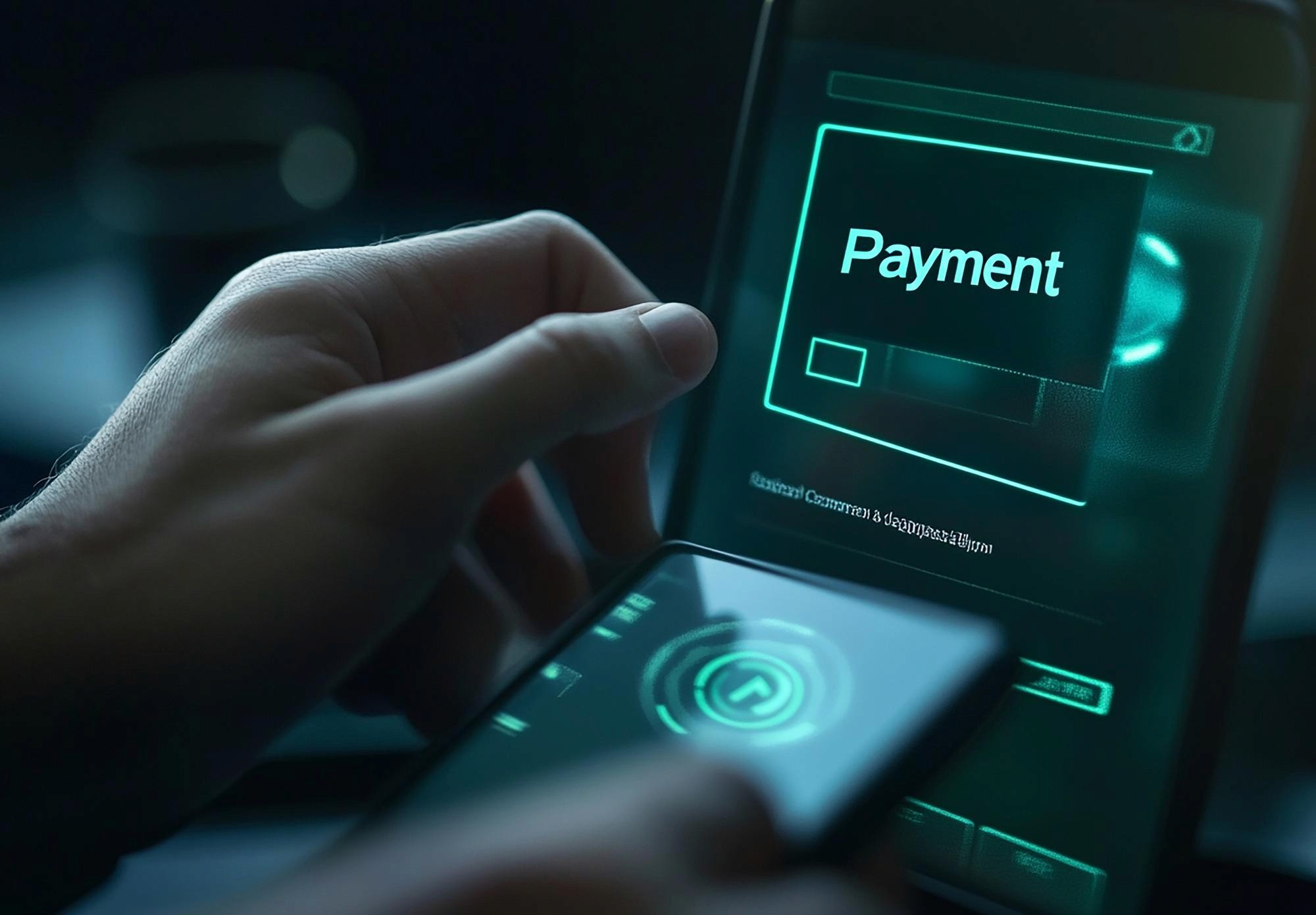
Watchlist Management
SEPA Instant Payments: Transforming Real-Time Transactions
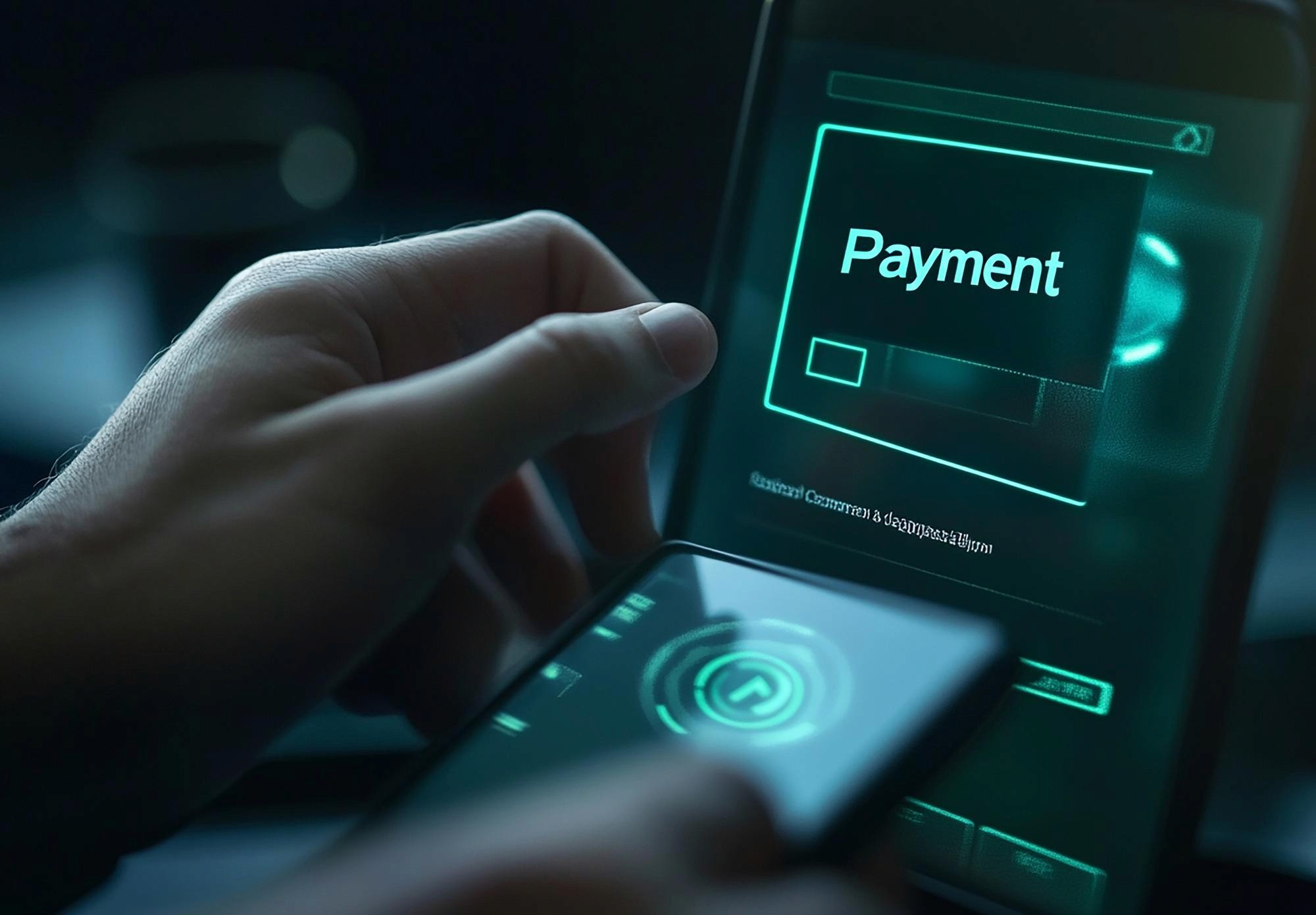
Watchlist Management
SEPA Instant Payments: Transforming Real-Time Transactions
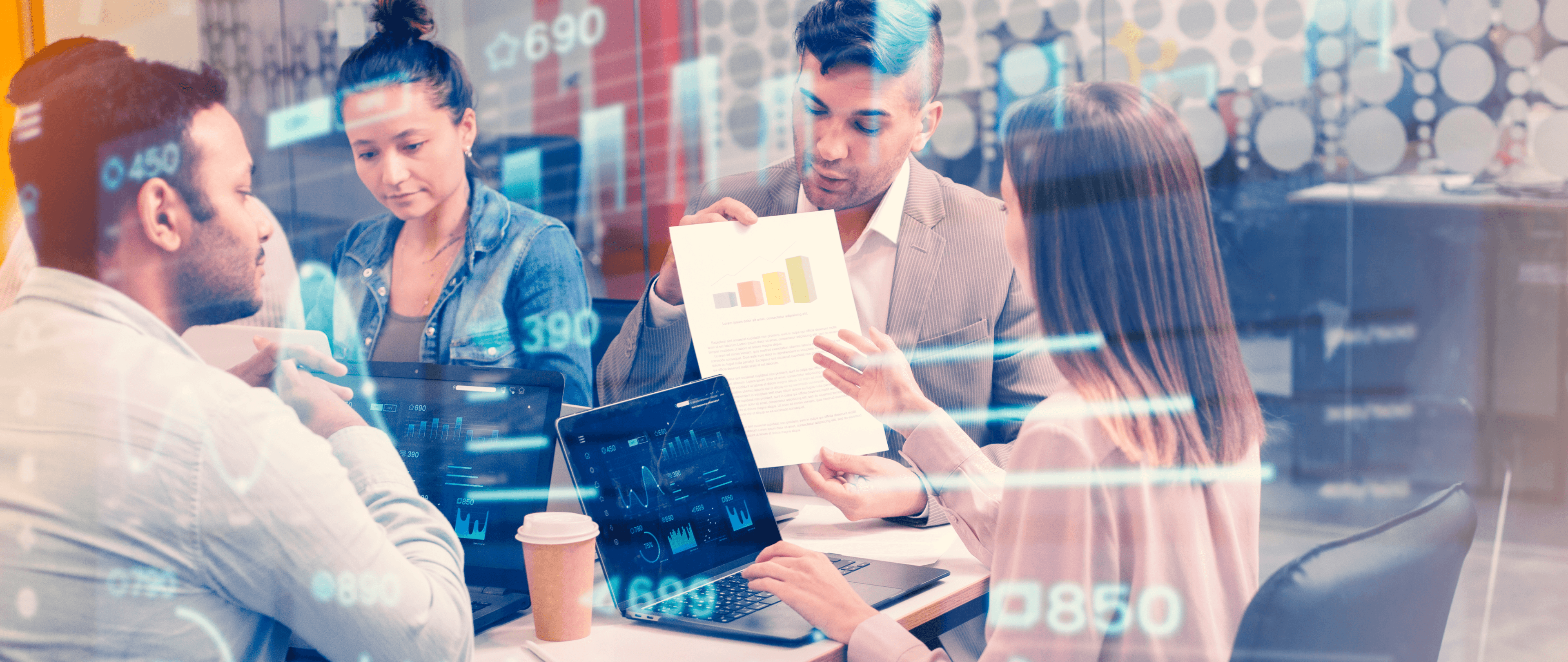
Sanctions Screening
Self-Assessments – Getting the Relationship Between the Firm and the Software Right
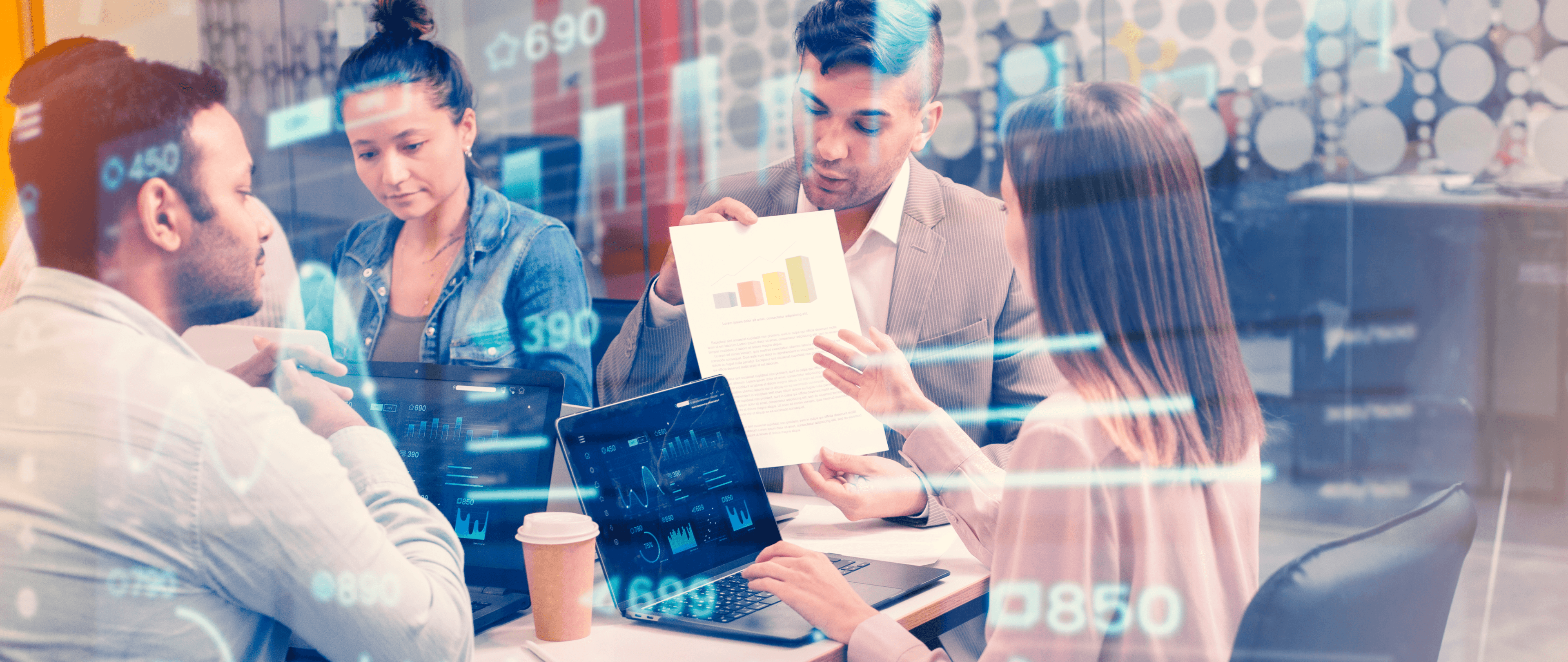
Sanctions Screening
Self-Assessments – Getting the Relationship Between the Firm and the Software Right
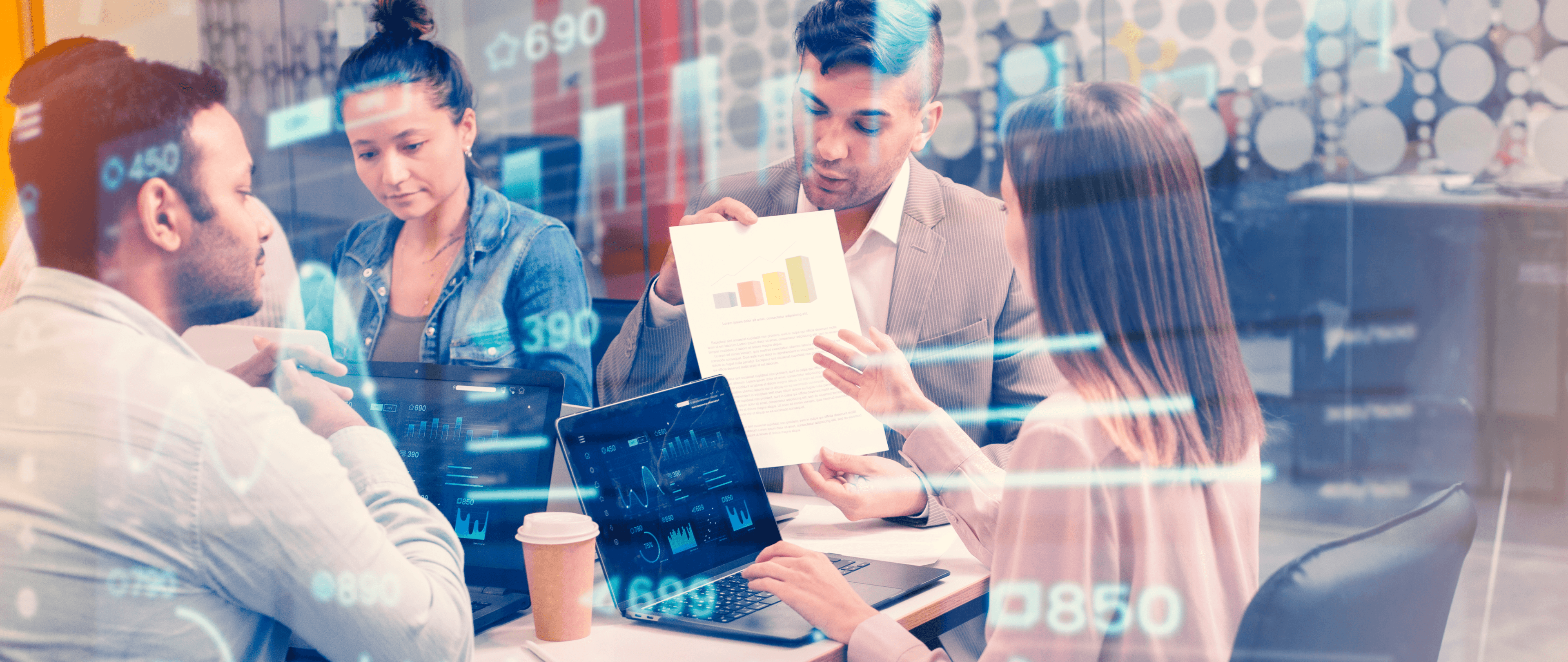
Sanctions Screening
Self-Assessments – Getting the Relationship Between the Firm and the Software Right
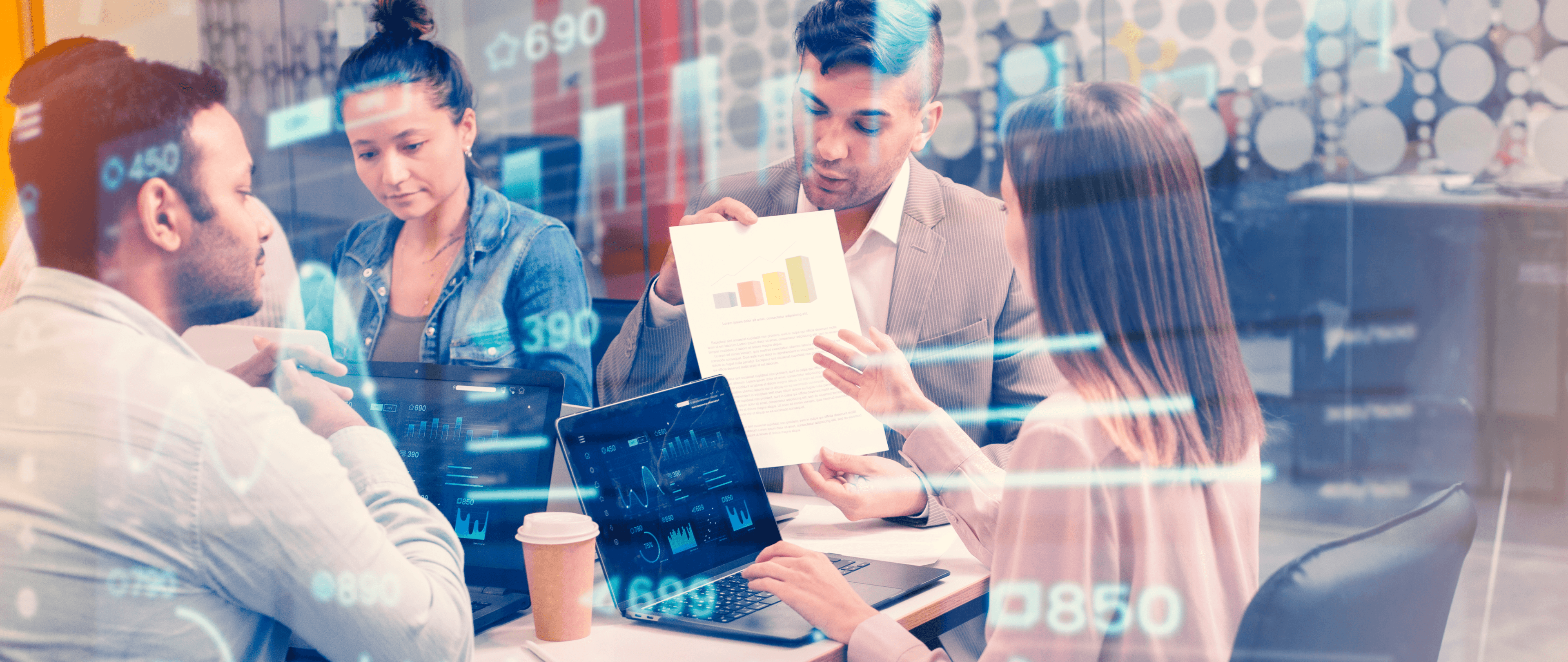
Sanctions Screening
Self-Assessments – Getting the Relationship Between the Firm and the Software Right
Sanctions Screening
Demystifying Sanctions Screening: 5 Critical Questions Every Financial Institution Must Answer
Sanctions Screening
Demystifying Sanctions Screening: 5 Critical Questions Every Financial Institution Must Answer
Sanctions Screening
Demystifying Sanctions Screening: 5 Critical Questions Every Financial Institution Must Answer
Sanctions Screening
Demystifying Sanctions Screening: 5 Critical Questions Every Financial Institution Must Answer
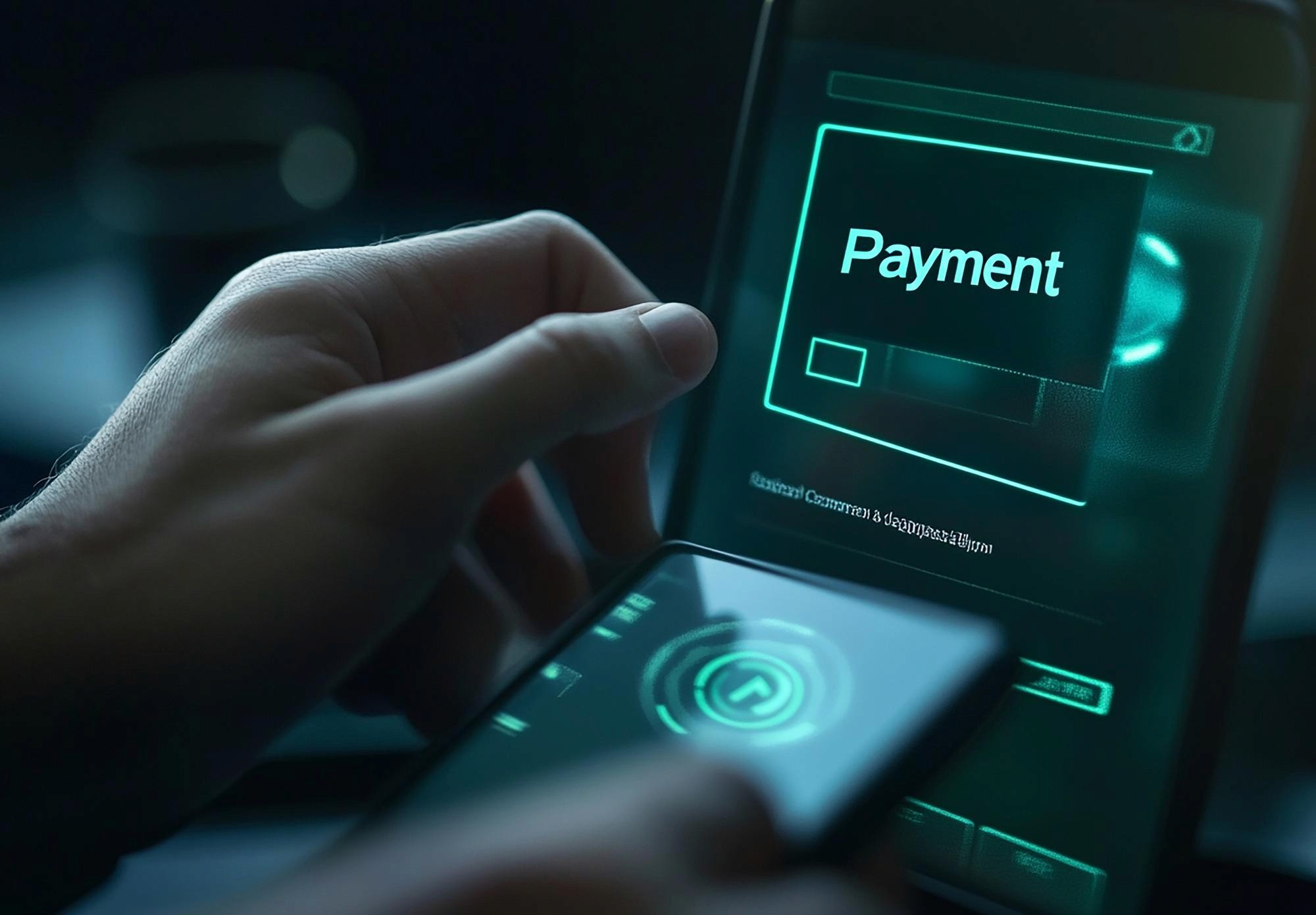
Watchlist Management
SEPA Instant Payments: Transforming Real-Time Transactions
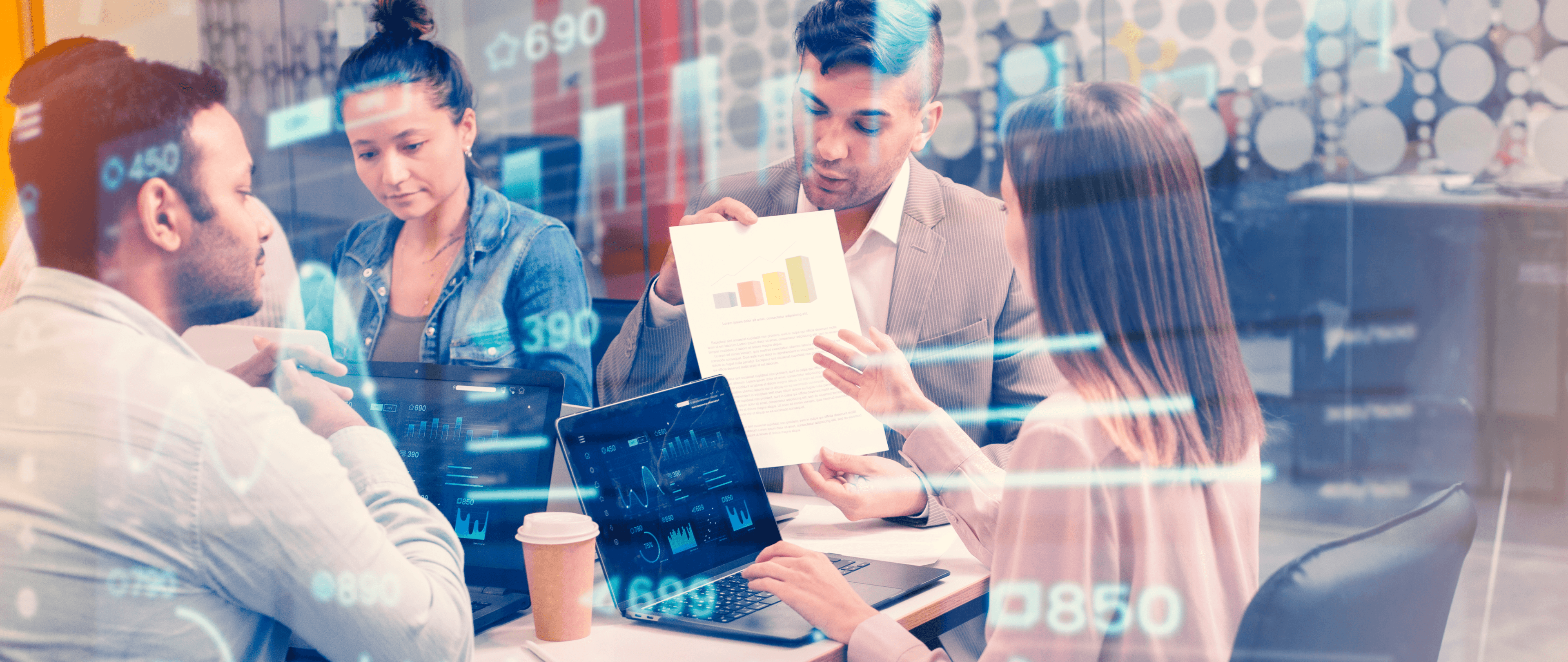
Sanctions Screening
Self-Assessments – Getting the Relationship Between the Firm and the Software Right
Sanctions Screening
Demystifying Sanctions Screening: 5 Critical Questions Every Financial Institution Must Answer
AML Compliance